The Ultimate Guide to Labelling Tool Machine Learning
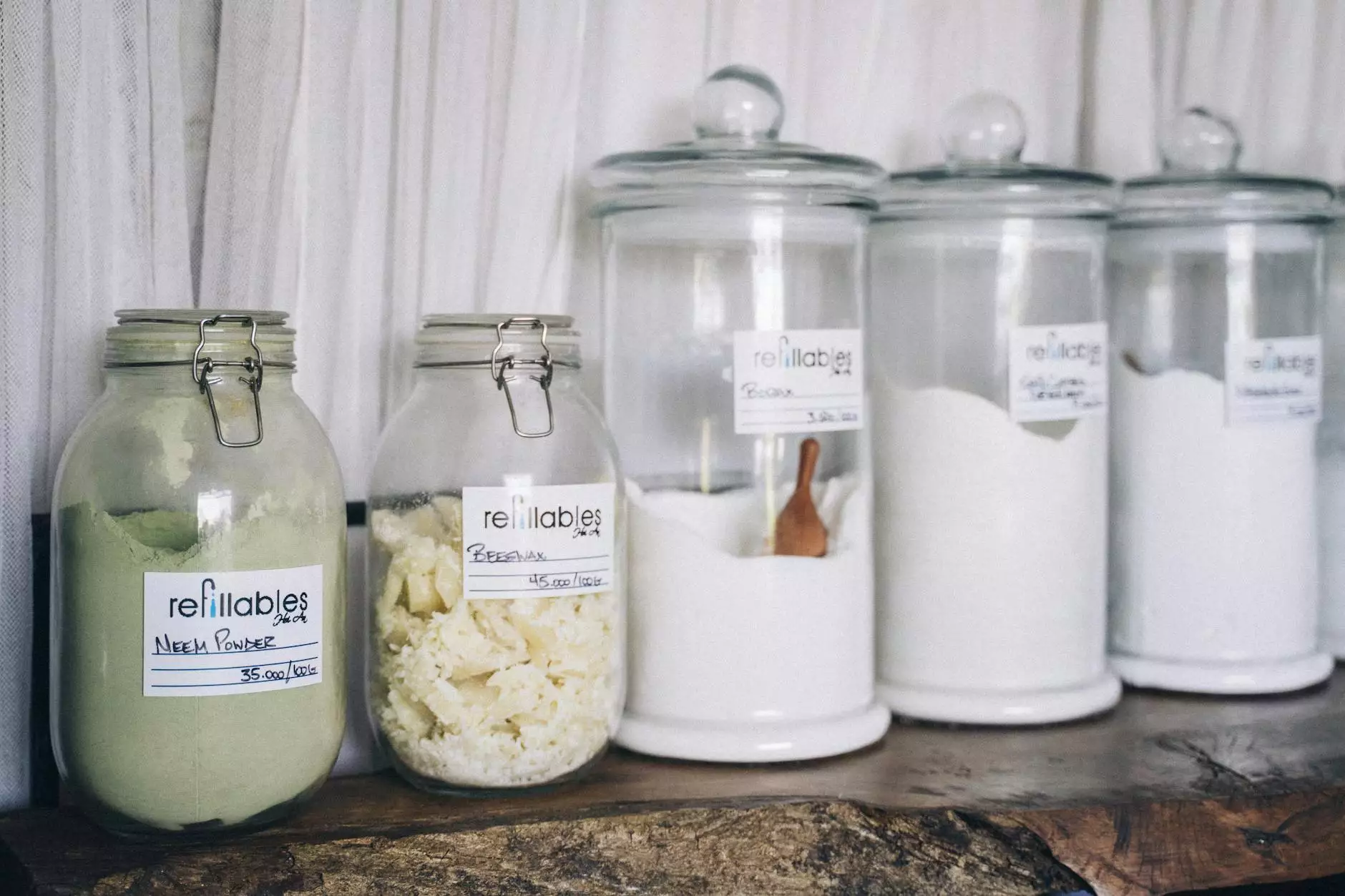
In the digital era where data drives business innovation and decision-making, comprehending the intricate world of machine learning is paramount. One key aspect that propels machine learning forward is the use of effective labelling tool machine learning techniques. This article delves deep into the significance of labelling in the machine learning process, particularly for businesses looking to leverage data annotation tools and platforms like Keylabs.ai.
Understanding Machine Learning and Data Annotation
Machine learning is a branch of artificial intelligence that enables systems to learn and improve from experience without being explicitly programmed. In this context, data annotation becomes crucial. Data annotation involves tagging or labelling data so that a machine learning model can learn from it. The accuracy and effectiveness of these models heavily depend on the quality of the labelled data.
The Role of Labelling Tools in Machine Learning
Effective labelling tools are essential for several reasons:
- Accuracy: High-quality labels lead to accurate models, which can perform better in prediction and classification tasks.
- Efficiency: Automated labelling tools significantly speed up the data preparation process, allowing businesses to focus on analysis rather than tedious manual tasks.
- Scalability: In today’s fast-paced world, the ability to scale data labelling operations is crucial, especially for companies handling vast amounts of data.
Components of an Exceptional Labelling Tool
A robust labelling tool should consist of various features that enhance the user experience, facilitate efficiency, and yield better results:
1. User-Friendly Interface
The user interface should be intuitive, allowing data annotators to navigate the tool easily while focusing on productivity rather than struggling with unnecessary complexity.
2. Customisation Options
Every business has specific needs. A good labelling tool must allow users to customise labelling types, workflows, and more.
3. Advanced Automation Features
Automation tools can significantly reduce the time required to label data. They can identify patterns, suggest labels, and even apply them in real-time.
4. Collaboration Capabilities
In many businesses, data labelling is a collaborative effort. A good labelling tool should facilitate team collaboration, enabling seamless communication and feedback.
The Impact of Labelling on Business Outcomes
The impact of using a reliable labelling tool machine learning can be transformative for businesses:
1. Enhanced Decision Making
When machine learning models are accurately trained with high-quality labelled data, businesses can make well-informed decisions based on precise predictions and insights.
2. Improved Customer Experiences
Businesses can leverage insights from machine learning models to enhance customer interactions, personalizing experiences and tailoring offerings to meet customer needs.
3. Efficient Operational Processes
By accelerating the data preparation process, labelling tools help companies streamline their operational workflows and allocate resources more effectively.
Choosing the Right Labelling Tool
When selecting a labelling tool, consider the following criteria:
- Cost-Effectiveness: The tool should provide value relative to its cost.
- Integration Capabilities: It should easily integrate with existing data systems and workflows.
- Support and Documentation: Reliable customer support and thorough documentation are essential for addressing issues and facilitating user onboarding.
Keylabs.ai: Leading the Way in Data Annotation
Keylabs.ai stands out as a premier provider of data annotation tools tailored for the machine learning domain. Their innovative platform offers:
1. High-Quality Data Annotation Services
The services provided by Keylabs.ai focus on quality, ensuring that every label meets the highest standards necessary for effective machine learning model training.
2. A Versatile Annotation Platform
From image and video annotation to text and audio data, Keylabs.ai accommodates a diversity of data types, making it suitable for various business needs.
3. Cutting-Edge Technology
The integration of machine learning in their labelling processes allows for advanced automation and intelligent labelling suggestions, enhancing efficiency and reducing human error.
Best Practices for Data Annotation in Machine Learning
Implementing effective data annotation strategies can differentiate successful businesses from their competitors:
1. Define Clear Labeling Guidelines
Establishing comprehensive and clear labelling guidelines helps maintain consistency across the dataset, which is critical for training accurate models.
2. Regularly Review and Update Labels
Regular audits and updates of labelled data ensure that the information remains relevant and accurate over time, which is important in dynamic environments.
3. Train Your Annotators
Providing proper training for your data annotators ensures they understand the nuances of the data and the importance of their work, contributing to higher quality outputs.
Conclusion: The Future of Labelling Tools in Machine Learning
As machine learning technology continues to evolve, the need for effective labelling tool machine learning solutions will only increase. Businesses that invest in high-quality data annotation tools, like those offered by Keylabs.ai, will be positioned to leverage their data for greater insights and improved decision-making capabilities. By understanding the significance of labelling in the machine learning process, companies can harness its full potential and drive their growth in a competitive landscape.
In summary, the value derived from high-quality labelled data cannot be overstated, and businesses seeking to excel in today's data-centric world must prioritize the integration of advanced labelling solutions.